Data Disaggregation & its key role in International Development
October 12, 2021
In the 2030 Agenda for Sustainable Development, the UN member states have pledged to leave no one behind – an unequivocal commitment to eradicate poverty, end discrimination and exclusion, and reduce the inequalities and vulnerabilities in all its forms. However, progress in this area has been slow. If we as a community are to truly reach the poorest of the poor and combat discrimination and rising inequalities, then concerted efforts are needed to boost the collection and use of reliable and high-quality data, disaggregated across multiple dimensions.
Our article is aimed at practitioners who work across NGOs, INGOs, governments, CSOs or other actors who are involved in development projects. The guide is intended to help development actors understand the concept of data disaggregation and inspire them to make it a part of every intervention. We will explore what data disaggregation is, how it’s different from data aggregation, its key benefits, common challenges, how it’s relevant for decision and policy making and why it’s important in international development.
What does Data Disaggregation mean?
Disaggregation of data refers to the breakdown of gathered information into smaller units or variables to gain a deeper understanding of a situation or to clarify underlying trends and patterns. Data may be grouped by different dimensions, such as age, sex, geographic area, education, ethnicity, disability, social status or other socioeconomic variables.
Disaggregation dimensions are the characteristics by which data is to be disaggregated (by sex, age, geographic location) and the disaggregation categories are the different characteristics under a certain disaggregation dimension (i.e: female/male).
According to the Inter-Agency and Expert Group on SDG indicators (IAEGSDGs),
“Disaggregation is the breakdown of observations within a common branch of a hierarchy to a more detailed level to that at which detailed observations are taken. With standard hierarchical classifications, categories can be split or disaggregated when finer details are required and made possible by the codes given to the primary observations.”
Aggregated data vs. disaggregated data
Before we explore data disaggregation further, let’s first understand how it differs from data aggregation.
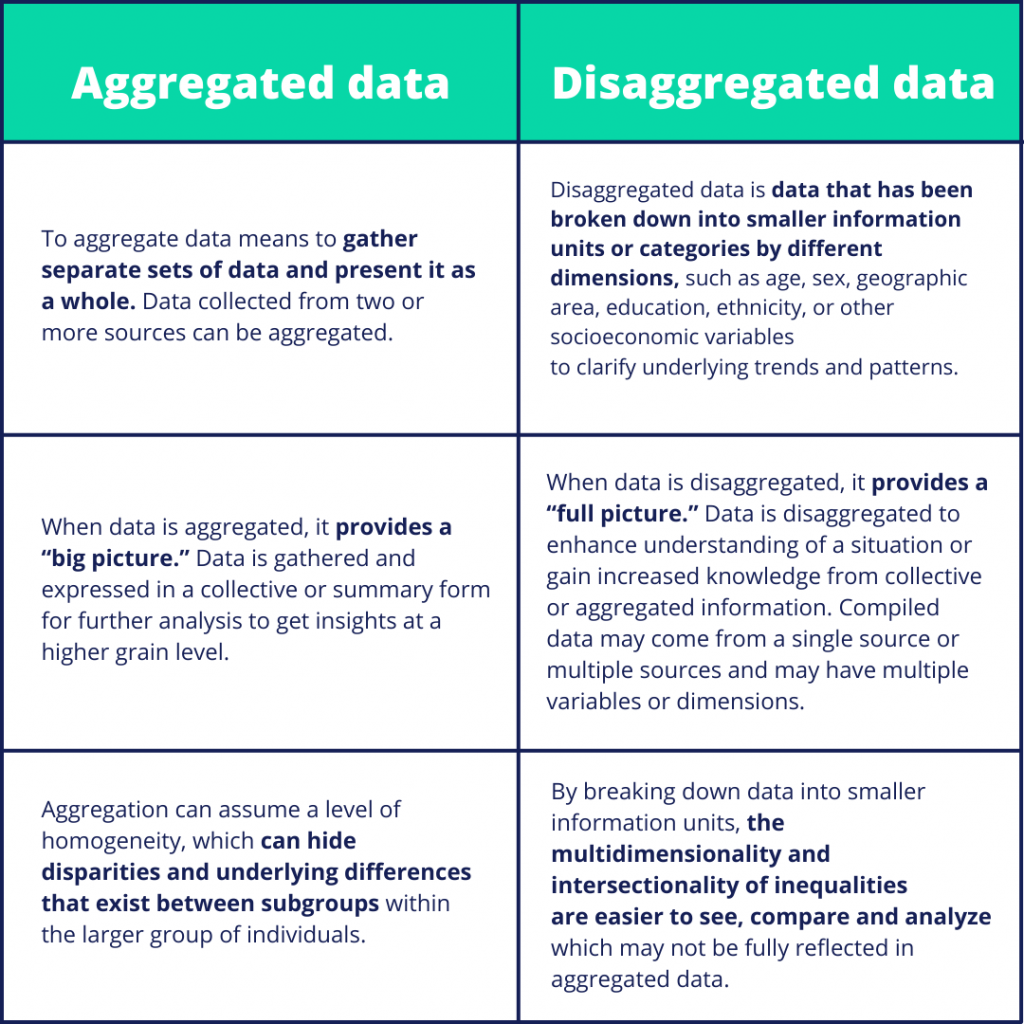
The above table shows the differences between the two concepts but it is important to keep in mind that even disaggregated data can be further aggregated by combining results from different activities, projects and programs to get a more comprehensive understanding of an organisation’s operations on a local, national or global level and to see its overall impact.
More on the aggregation of disaggregation data and how TolaData can assist you with the process.
Benefits of Data Disaggregation for the International Development community
Data disaggregation is critical to development success and sustainability. By disaggregating data into many variables, policymakers, donors, community leaders and beneficiaries are better equipped to understand their challenges and opportunities which fosters informed decision and policymaking, ultimately promoting a just and equitable distribution of resources. These decisions in turn can help create appropriate and effective interventions for more equitable outcomes for community members across different dimensions.
According to the United Nations Statistics Division (UNSD), data disaggregation is now also one of the nine pillars of the “Data Revolution.” Data disaggregation helps to improve the quality and availability of statistics on local, national and global levels – high quality, reliable, accessible, timely and open disaggregated data addresses key data gaps and adds valuable information to the evidence base for future analysis and research.
Here’s the summary of the common benefits of disaggregating data into several relevant sub-units or variables:
- Data disaggregation portrays a more holistic picture of an intervention. By providing precise understanding, definition, characteristics of the target group and the nuances, complexities, trends, patterns and links within a project, its activities and outcomes, data disaggregation helps you to detect and analyse problems and needs more accurately.
- Data disaggregation helps to pinpoint populations with specific needs. Data Disaggregation helps you to see beyond just the number of people you have reached through your project. It allows you to dive deeper to see their characteristics, like their age, gender, location, income level and other variables that you may choose to disaggregate by. It allows for routine comparisons and in-depth trend and characteristic analysis across different target groups and sub-groups. This ultimately helps to shape projects and policies to support the advancement of a community or a nation’s collective well-being and ensure sustainable development.
- Disaggregation of data is an important way to ensure inclusiveness. Disaggregation allows more detailed data analysis to identify inequalities, making the issues and the voices of marginalized and vulnerable populations more visible to policymakers and stakeholders which makes it possible for an organisation to focus its response where it’s needed most.
- Disaggregated data is key to effective policymaking and interventions because it provides enough evidence for the development actors to understand what works for one demographic group may not work for another. Different people face different constraints depending on their gender, age, income, location, and other factors, production systems, education levels etc. This enables the development actors and policymakers to advocate for more tailored, effective and efficient policies and interventions that align with the different groups and subgroups’ needs, concerns and circumstances.
- Disaggregated data is also critical in the analysis of project indicators which is key in determining the actual progress of a project against its set targets and critical goals – this helps the project team to make the necessary changes to the design and implementation of their projects to maximize their impact and outcomes.
- Data disaggregation helps you to see your overall impact. When you choose to aggregate your data results across multiple projects and programs, it harmonizes data on multiple levels and across diverse themes, enabling you to see, for example, your organisation’s overall impact across multiple dimensions. This helps you to determine whether or not your projects are creating the intended change or impact.
Let’s take a simple example…
Let’s say, you designed two surveys to collect data from 400 members in a community to measure the average income. In your first survey, you only include a few questions: the names of their respondents, total household income or income of a specific family member, expenses etc. The result you are going to get from this survey will reflect the average income in that community which will get your job done but it doesn’t provide any additional insights.
Whereas, in your second survey you break down your question into smaller units, like including their age group, the gender of the respondents, their ethnicity, the location of the respondents, the years of their employment, their educational levels and backgrounds, the nature of their employment, how satisfied they are with their jobs etc. then the insight you are going to derive from this survey will be much richer than the first one.
The second survey will not only show the average income in that community but will also reflect more complexity, trends and issues related to the income generation process within that community, and highlight the characteristics of the respondents and many additional factors that play a crucial role in shaping the economy of that community, which will ultimately help you design or adapt your project in a better manner to mitigate the issues and target the underlying problems. For example, by disaggregating the average income by gender, you can clearly see the stark disparity in income levels between the male and female respondents in that community and so on.
Is there a minimum set of suggested disaggregation?
Data can be disaggregated into various dimensions but the minimum disaggregation types are determined by the objectives of your intervention, the indicators and targets set in your results framework and your reporting and donor requirements, so it varies on a case by case basis. However, the UN Fundamental Principles of Official Statistics states that all standard indicators can be and should be disaggregated at least by gender, age, ethnic origin, disability, income and geographic location.
Before you select the disaggregation variables, make sure you understand your data requirements – what information can help you understand the needs of the target groups and analyse your outcomes and impact better and how are you going to collect them? Which disaggregation variables will help you meet those data needs? Thinking about your data needs beforehand will help you set the most appropriate disaggregation questions for your survey which will help you generate rich data and insights.
Below is the summary of the data disaggregation principles set by the Global Partnership for Sustainable Development which can help you get a head start with the disaggregation process:
- First and foremost, identify the objective and scope for disaggregating your data.
- All target populations must be included in the data because we can only achieve the “leave no one behind” goal by empowering the furthest behind.
- Remember, all data should, wherever possible, be disaggregated in order to accurately describe the target populations.
- Data should be drawn from all available sources for a comprehensive understanding of the realities on the ground.
- Use both qualitative and quantitative data. (More on Qualitative vs. Quantitative data.)
- Those responsible for the collection of data and production of statistics must be ethical and accountable.
- Human and technical capacity to collect, analyse, and use disaggregated data must be improved where needed, through adequate and sustainable financing. Collecting and analyzing disaggregated data needs specific skills and these must be built.
- Boost the use of technology that supports disaggregation. (See how TolaData can help.)
- Don’t forget about data privacy and protection, your respondents must feel safe at all times. Confidentiality and privacy must be maintained to ensure personal data is not abused, misused, or putting anyone at risk of identification or discrimination.
Source: Adapted from Global Partnership for Sustainable Development Data. Inclusive Data Charter vision and principles.
Challenges in data disaggregation
Data disaggregation may look different across diverse contexts. However, there are a few key issues and some overarching challenges that may arise during data disaggregation within any project.
The most common challenge comes with data privacy and protection related to disaggregated data. Appropriate data protection architecture is necessary for maintaining the confidentiality of respondents’ information through all stages of the data cycle. Any potential risks to the respondent should be avoided if possible or addressed in a timely manner.
Some projects choose population census or other secondary data as one of the data sources but you must be wary that in many parts of the world population census is updated approx. once every decade, so the data you are collecting might not be accurate, timely and 100% reliable. Plus, the census data or other public statistics (e.g. ministry surveys) often lack the information on sub-groups or the disaggregation details you need. The ideal solution would be to collect data from multiple sources and combining secondary data with primary data and triangulating them. Data triangulation allows for verification and comparison of data as well as captures different dimensions of the same phenomenon.
In addition, there are other practical challenges that obstruct progress in disaggregation. In some bigger projects, disaggregation requires more intensive data collection, management and analysis and it could be technically difficult or very costly, so many organisations may not choose to prioritize it. Other challenges include limited coordination among the team and other involved parties, lack of awareness of the importance of disaggregated data, or limited knowledge, capacity and resources.
Data disaggregation is a critical step towards achieving sustainable development and identifying and addressing the needs of the target groups, mostly of the vulnerable and marginalized communities. Collection and analysis of high-quality disaggregation data can provide a solid evidence base for use by governments, the private sector, academic researchers, and others to advance equality and economic and social development.
However, disaggregating data can be challenging in practice due to the lack of resources, knowledge and capacity. But on the bright side, efforts continue on a national and global level to develop relevant data disaggregation tools, frameworks, guidance & resources for practitioners. In the meantime, all individuals, organisations and countries working in disaggregation must collaborate with each other as much as possible to discuss and share experiences, good practices and lessons learned.
We hope you found our article helpful. If you have any recommendations or suggestions for improvement, then please feel free to mention them in the comment section below.
Key References
- Disaggregated data is essential to leave no one behind; IISD
- Why data disaggregation is key during a pandemic; PAHO, WHO
- The importance of disaggregated data; National Collaborating Center for Aboriginal Health
- Leave no migrant behind, the 2030 agenda and data disaggregation; IOM
- Practical guidebook on data disaggregation for the sustainable development goals, ADB
- Data Disaggregation and the Global Indicator Framework, United Nations Statistical Division
By Chandani Lopez Peralta, Content Marketing Manager at TolaData.